Data Mining for Targeted Marketing
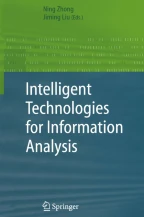
Targeted marketing is a new business model of interactive one-to-one communication between marketer and customer. There is great potential for data mining to make useful contributions to the marketing discipline for business intelligence. This chapter provides an overview of the recent development in data mining applications for targeted marketing.
This is a preview of subscription content, log in via an institution to check access.
Access this chapter
Subscribe and save
Springer+ Basic
€32.70 /Month
- Get 10 units per month
- Download Article/Chapter or eBook
- 1 Unit = 1 Article or 1 Chapter
- Cancel anytime
Buy Now
Price includes VAT (France)
eBook EUR 117.69 Price includes VAT (France)
Softcover Book EUR 158.24 Price includes VAT (France)
Hardcover Book EUR 158.24 Price includes VAT (France)
Tax calculation will be finalised at checkout
Purchases are for personal use only
Preview
Similar content being viewed by others
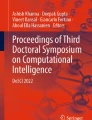
Machine Learning and Data Mining Use Cases in the Development of Marketing Strategies
Chapter © 2023
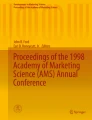
An Overview of Data Mining and Marketing
Chapter © 2015
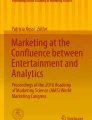
Special Session: Big Data Analytics for Marketing (Contributed Session by the IÉSEG Center for Marketing Analytics (ICMA))
Chapter © 2017
References
- R. Agrawal, T. Imielinski, A. Swami: Mining Association Rules Between Sets of Items in Large Aatabases. Proc. ACM SIGMOD International Conference on the Management of Data (ACM Press, 1993 ) pp. 207–216 Google Scholar
- R. Agrawal, H. Mannila, R. Srikant, H. Toivonen, A.I. Verkamo: Fast Discovery of Association Rules, Advances in Knowledge Discovery and Data Mining (MIT Press, 1996 ) pp. 307–328 Google Scholar
- L. Breiman, J.H. Friedman, R.A. Olshen, C.J. Stone: Classification and Regression Trees (Wadsworth, 1984 ) Google Scholar
- David Shepard Associates: The New Direct Marketing (McGraw-Hill, 1999) Google Scholar
- F. Berman: From TeraGrid to Knowledge Grid, CACM, 44, 27–28 (2001) Google Scholar
- M. Cannataro and D. Talia: The Knowledge Grid, CA CM, 46, 89–93 (2003) Google Scholar
- G. Dong and J. Li: Efficient Mining of Emerging Patterns: Discovering Trends and Differences. Proc. 5th ACM SIGKDD International Conference on Knowl-edge Discovery and Data Mining (KDD-99) (ACM Press, 1999 ) pp. 43–52 Google Scholar
- P.C. Fishburn: Seven Independence Concepts and Continuous Multiattribute Utility Functions. Journal of Mathematical Psychology, 11, 294–327 (1974) ArticleMathSciNetMATHGoogle Scholar
- I. Foster and C. Kesselman: The Grid: Blueprint for a New Computing Infras-tructure (Morgan Kaufmann, 1999 ) Google Scholar
- I. Foster and C. Kesselman: The Grid 2: Blueprint for a New Computing Infrastructure (Morgan Kaufmann, 2004 ) Google Scholar
- J. Han, Y. Cai, N. Cercone: Data-Driven Discovery of Quantitative Rules in Relational Databases. IEEE Transaction on Knowledge and Data Engineering, 5, 29–40 (1993) ArticleGoogle Scholar
- J. Han, M. Kamber: Data Mining: Concepts and Techniques (Morgan Kaufmann, 2001 ) Google Scholar
- S.Y. Hwang, E.P. Lim, J.H. Wang, J. Srivastava: Proc. PAKDD 2002 Workshop on Mining Data across Multiple Customer Touchpoints for CRM (2002) Google Scholar
- J.J. Jonker, P.H. Franses, N. Piersma: Evaluating Direct Marketing Campaigns; Recent Findings and Future Research Topics. Erasmus Research Institute of Management (ERIM), Erasmus University Rotterdam in Its Series Discussion Paper with Number 166 (2002) Google Scholar
- W. Klosgen, J.M. Zytkow: Handbook of Data Mining and Knowledge Discovery (Oxford University Press, 2002 ) Google Scholar
- S. Kullback and R.A. Leibler: On Information and Sufficiency. Annals of Mathematical Statistics, 22, 79–86 (1951) ArticleMathSciNetMATHGoogle Scholar
- D.B. Leake: Case-Based Reasoning (AAAI Press, 1996) Google Scholar
- C.X. Ling, C. Li: Data Mining for Direct Marketing: Problems and Solutions. Proc. 4th International Conference on Knowlege Discovery and Data Mining (KDD’98) (AAAI Press, 1998 ) pp. 73–79 Google Scholar
- J. Nabrzyski, J.M. Schopf, J. Weglarz: Grid Resource Management (Kluwer, 2004 ) Google Scholar
- Z. Pawlak: Rough Sets, Theoretical Aspects of Reasoning about Data (Kluwer, 1991 ) Google Scholar
- P. Van Der Putten: Data Mining in Direct Marketing Databases, W. Baets (ed.) Complexity and Management: A Collection of Essays (World Scientific, 1999 ) Google Scholar
- R. Potharst, U. Kaymak, W. Pijls: Neural Networks for Target Selection in Direct Marketing, In K.A. Smith, J.N.D. Gupta (eds.) Networks in Business: Techniques and Applications (Idea Group Publishing, 2001 ) Google Scholar
- J.R. Quinlan: Programs for Machine Learning (Morgan Kaufmann, 1993)130 N. Zhong et al. Google Scholar
- C.R. Rao: Diversity and Dissimilarity Coefficients: a Unified Approach. Theoretical Population Biology, 21, 24–43 (1982) ArticleMathSciNetMATHGoogle Scholar
- B. Ratner: Finding the Best Variables for Direct Marketing Models. Journalof Targeting Measurement and Analysis for Marketing, 9, 270–296 (2001) ArticleGoogle Scholar
- S.E. Robertson: On Relevance Weight Estimation and Query Expansion. Jour-nal of Documentation, 42, 182–188 (1986) ArticleGoogle Scholar
- S.E. Robertson, K. Sparck Jones: Relevance Weighting of Search Terms. Jour-nal of the American Society for Information Science, 27, 129–146 (1976) ArticleGoogle Scholar
- Y. Sai, Y.Y. Yao, N. Zhong: Data Analysis and Mining in Ordered Information Tables. Proc. 2001 IEEE International Conference on Data Mining (ICDM’01) (IEEE Computer Society Press, 2001 ) pp. 497–504 Google Scholar
- G. Salton and M.H. McGill: Introduction to Modern Information Retrieval (McGraw-Hill, 1983 ) Google Scholar
- E. Suzuki: Autonomous Discovery of Reliable Exception Rules, Proc Third International Conference on Knowledge Discovery and Data Mining (KDD-97) (AAAI Press, 1997) pp. 259–262 Google Scholar
- K.S. Jones, P. Willett: Readings in Information Retrieval (Morgan Kaufmann, 1997 ) Google Scholar
- A.R. Simon, S.L. Shaffer: Data Warehousing and Business Intelligence for e-Commerce (Morgan Kaufmann, 2001 ) Google Scholar
- J. Srivastava, R. Cooley, M. Deshpande, P. Tan: Web Usage Mining: Discovery and Applications of Usage Patterns from Web Data. SIGKDD Explorations, Newsletter of SIGKDD, 1, 12–23 (2000) ArticleGoogle Scholar
- R. Stone: Successful Direct Marketing Methods, 6th ed. (NTC Business Books, 1996 ) Google Scholar
- D. Van den Poel, Z. Piasta: Purchase Prediction in Database Marketing with the ProbRough System, In L. Polkowski, A. Skowron (eds.) Rough Sets and Current Trends in Computing, LNAI 1424, 593–600 (Springer, 1998 ) Google Scholar
- S. Watanabe: Pattern Recognition as a Quest for Minimum Entropy. Pattern Recognition, 13, 381–387 (Elsevier, 1981 ) Google Scholar
- M. Wedel, W.A. Kamakura: Market Segmentation: Conceptual and Methodological Foundations (Kluwer, 1999 ) Google Scholar
- S.K.M. Wong, Y.Y. Yao: A Probability Distribution Model for Information Retrieval. Information Processing and Management, 25, 39–53 (1989) ArticleGoogle Scholar
- S.K.M. Wong, Y.Y. Yao: A Generalized Binary Probabilistic Independence Model. Journal of the American Society for Information Science, 41, 324–329 (1990) ArticleGoogle Scholar
- S.K.M. Wong, Y.Y. Yao: An Information-Theoretic Measure of Term Specificity. Journal of the American Society for Information Science, 43, 54–61 (1992) ArticleGoogle Scholar
- Y.Y. Yao, S.K.M. Wong, C.J. Butz: On Information-Theoretic Measures of Attribute Importance. In N. Zhong, L. Zhou (eds.) Methodologies for Knowledge Discovery and Data Mining, LNAI 1574 (Springer, 1999 ) pp. 479–488 Google Scholar
- Y.Y. Yao, N. Zhong: Mining Market Value Functions for Targeted Marketing. Proc. 25th IEEE International Computer Software and Applications Conference (COMPSAC’01) (IEEE Computer Society Press, 2001 ) pp. 517–522 Google Scholar
- Y.Y. Yao, N. Zhong: Granular Computing Using Information Tables. T.Y. Lin, Y.Y. Yao, L.A. Zadeh (eds.) Data Mining, Rough Sets and Granular Computing (Physica-Verlag, 2002 ) pp. 102–124 Google Scholar
- Y.Y. Yao, N. Zhong, J. Huang, C. Ou, C. Liu: Using Market Value Functions for Targeted Marketing Data Mining. International Journal of Pattern, Recognition and Artificial Intelligence, 16 (8) 1117–1131 (World Scientific, 2002 ) Google Scholar
- N. Zhong, J.Z. Dong, C. Liu, S. Ohsuga: A Hybrid Model for Rule Discovery in Data. Knowledge Based Systems,14 (7) 397–412 (Elsevier, 2001) 6. Data Mining for Targeted Marketing 131 Google Scholar
- N. Zhong, S. Ohsuga: Automatic Knowledge Discovery in Larger Scale Knowledge-Data Bases. In C. Leondes (ed.) the Handbook of Expert Systems, 4, 1015–1070 (Academic Press, 2001 ) Google Scholar
- N. Zhong, C. Liu, S. Ohsuga: Dynamically Organizing KDD Processes, International Journal of Pattern Recognition and Artificial Intelligence, 15 (3) 451–473 (World Scientific, 2001 ) Google Scholar
- N. Zhong, Y.Y. Yao, M. Ohshima, S. Ohsuga: Interestingness, Peculiarity, and Multi-Database Mining. Proc. 2001 IEEE International Conference on Data Mining (ICDM’01) (IEEE Computer Society Press, 2001 ) pp. 566–573 Google Scholar
- N. Zhong, J.Z. Dong, S. Ohsuga: Using Rough Sets with Heuristics to Feature Selection. Journal of Intelligent Information Systems, 16 (3) 199–214 (Kluwer, 2001 ) Google Scholar
- N. Zhong, A. Skowron: A Rough Sets Based Knowledge Discovery Process. International Journal of Applied Mathematics and Computer Science, 11 (3) 101–117 (Technical University Press, 2001 ) Google Scholar
- N. Zhong, J. Liu, Y.Y. Yao (eds.): In Search of the Wisdom Web, IEEE Computer, 35 (11) 27–31 (2002) Google Scholar
- N. Zhong, J. Liu, Y.Y. Yao (eds.): Web Intelligence (Springer, 2003 ) Google Scholar
- N. Zhong: Toward Web Intelligence. In E.M. Ruiz, J. Segovia, P.S. Szczepaniak(eds.) Advances in Web Intelligence, LNAI 2663 (Springer, 2003 ) pp. 1–14 Google Scholar
- N. Zhong, Y.Y. Yao, M. Ohshima: Peculiarity Oriented Multi-Database Min-ing, IEEE Transaction on Knowlegde and Data Engineering, 15 (4) 952–960 (2003) Google Scholar
- N. Zhong: Developing Intelligent Portals by Using WI Technologies, Proc. the Second International Conference on Active Media Technology (AMT’04) (World Scientific, 2004) Google Scholar
Author information
Authors and Affiliations
- Maebashi Institute of Technology, Japan Ning Zhong
- University of Regina, Canada Yiyu Yao
- Beijing University of Technology, China Chunnian Liu, Jiajin Huang & Chuangxin Ou
- Ning Zhong